B08 - Continuous learning by integrating reinforcement learning and data assimilation to individualise drug treatments
This project started with the second funding period in July 2021.
Objectives
Understanding the basis of variability in the response of patients to drug treatment is one of the key challenges in drug therapy. Some patients respond well and others less so; some develop adverse events even at low doses, whereas others could probably tolerate and gain additional benefit from higher doses than they receive.
Precision dosing focuses on the individualisation of drug treatment based on patient factors known to alter drug disposition and/or response. It is often combined with therapeutic drug/biomarker monitoring, i.e., measuring drug or biomarker concentrations of a patient regularly in time. With few exceptions (e.g., continuous monitoring on the intensive care unit), the typical scenario is data sparse in time and with limited ability to observe the system.
An important field of application is in cytotoxic chemotherapy, where the presence of a narrow therapeutic window and large inter-individual variability indicate individualised drug regimes for safe and efficacious therapies. A typical scenario comprises a few blood samples within each of the six three-weekly cycles.
Based on the complexity of the biological processes involved and the larger number of treatment options, model-informed precision dosing (MIPD) has gained considerable attention. It builds on mathematical models of the drug-patient-disease system, often describing the pharmacokinetics, pharmacodynamics, toxicity and responses of the drug(s). A well-founded and generally applicable framework to continuously learn from patient-specific data in a MIPD context, for an individual patient over the course of the therapy or across patients with the same therapy, however, is still lacking.
This project aims at a novel class of model-informed precision dosing approaches based on a combination of reinforcement learning (RL) and sequential data assimilation (DA) with a focus on the sparse data setting. We have recently demonstrated first promising results in two studies in the context of cytotoxic chemotherapy [1,2]. These are the merits of a fruitful collaboration within the CRC and an associated project on data assimilation in systems pharmacology. While we have demonstrated a proof-of-concept, there exist major challenges and open issues that need to be addressed, in particular to have a wide impact in the clinical domain.
[1] C. Maier and N. Hartung and J. de Wiljes and C. Kloft and W. Huisinga (2020) Bayesian data assimilation to support informed decision-making in individualized chemotherapy CPT: Pharmacometrics & Systems Pharmacology 9(3), 153–164, https://pubmed.ncbi.nlm.nih.gov/31905420/.
[2] C. Maier and N. Hartung and C. Kloft and W. Huisinga and J. de Wiljes (2021) Reinforcement learning and Bayesian data assimilation for model-informed precision dosing in oncology. CPT: Pharmacometrics & Systems Pharmacology10(3):241-254, doi: 10.1002/psp4.12588.
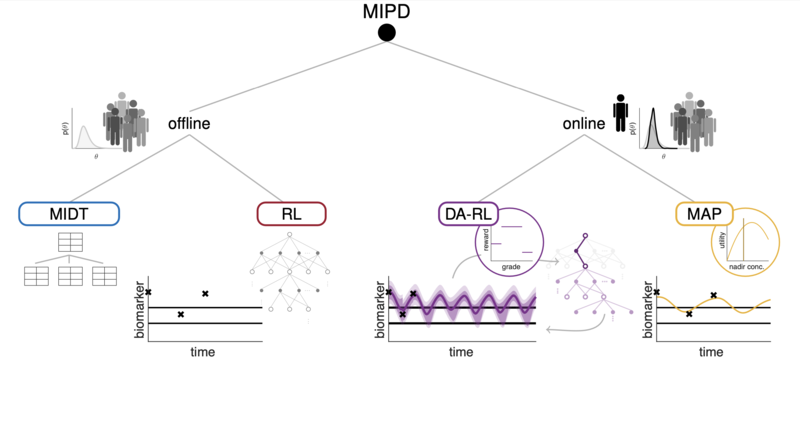