B09 - Neural network modelling of brain responses during language comprehension
This project started with the second funding period in July 2021.
Objectives
Language processing is one of the most intriguing human capacities. Recent developments in artificial intelligence show that artificial neural networks (deep learning models) perform increasingly well on complex tasks including language comprehension. A rapidly evolving research program at the interface of artificial intelligence and cognitive neuroscience focuses on the question of in how far these artificial neural networks can serve as models of corresponding human capacities. Here, we specifically address the issue of in how far deep learning language models provide good models of human language comprehension.
We use a scaled-up version of the Sentence Gestalt (SG) model (see figure above from Rabovsky et al, Nature Human Behavior, 2018), a neural network model of sentence comprehension that we used in previous work to simulate a language related brain response (the N400 component of the event related brain potential, ERP). In the proposed project, we aim to investigate whether and how activation states in the large-scale model's hidden layer can predict the spatio-temporal dynamics of neural activation in the brain's language network as measured by magnetencephalography (MEG) and electrocorticography (ECoG). We will compare the model's fit to neural data with other deep learning language models. Furthermore, we will also explore Bayesian derivative-free correlation-based learning algorithms for model training, and will compare the models' capacity to account for neural data between models trained via different algorithms. As a long-term goal, we hope that the investigation of correspondences between neural network language models trained using Bayesian approaches and brain data obtained during language comprehension may contribute to the debate concerning in how far the brain relies on Bayesian computation.
The project will closely interact with projects A06 on Bayesian inference and project B03 on cognitive modelling.
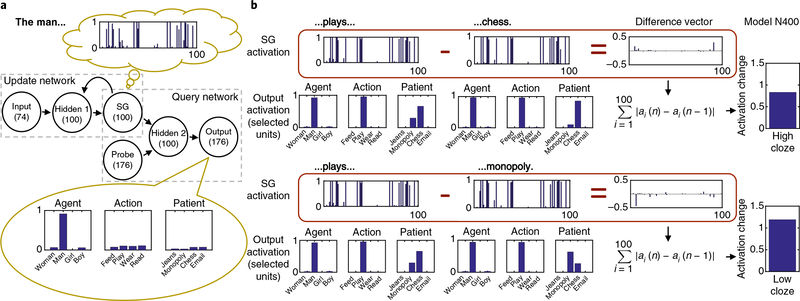
Preprints
Lopopolo, A. and Rabovsky, M. (2023). Tracking lexical and semantic prediction error underlying the N400 using artificial neural network models of sentence processing. doi: 10.1101/2022.11.14.516396
Bhandari, D., Pidstrigach, J., and Reich, S. (2023). Affine Invariant Ensemble Transform Methods to Improve Predictive Uncertainty in ReLU Networks. arXiv:2309.04742
Publications
Bhandari, D., Pidstrigach, J., and Reich, S. (2024): Affine Invariant Ensemble Transform Methods to Improve Predictive Uncertainty in ReLU Networks, Foundations of Data Science, Foundations of Data Science, published online doi:10.3934/fods.2024040, arXiv:2309.04742
Lopopolo, A. and Rabovsky, M. (2024): Tracking lexical and semantic prediction error underlying the N400 using artificial neural network models of sentence processing, Neurobiology of Language, 5 (1): 136–166, doi:10.1162/nol_a_00134, bioRxiv 2022.11.14.516396
Pidstrigach, J. and Reich, S. (2022). Affine-invariant ensemble transform methods for logistic regression. Foundation of Computational Mathematics, 22. doi:10.10007/s10208-022-09550-2.