Welcome to the collaborative Research Center TRR 181 ”Energy transfers in Atmosphere and Ocean“
The seamless integration of large data sets into sophisticated computational models provides one of the central research challenges for the mathematical sciences in the 21st century. When the computational model is based on evolutionary equations and the data set is time-ordered, the process of combining models and data is called data assimilation. The assimilation of data into computational models serves a wide spectrum of purposes ranging from model calibration and model comparison all the way to the validation of novel model design principles.
The field of data assimilation has been largely driven by practitioners from meteorology, hydrology and oil reservoir exploration; but a theoretical foundation of the field is largely missing. Furthermore, many new applications are emerging from, for example, biology, medicine, and the neurosciences, which require novel data assimilation techniques. The goal of the proposed CRC is therefore twofold: First, to develop principled methodologies for data assimilation and, second, to demonstrate computational effectiveness and robustness through their implementation for established and novel data assimilation application areas.
While most current data assimilation algorithms are derived and analyzed from a Bayesian perspective, the CRC will view data assimilation from a general statistical inference perspective. Major challenges arise from the high-dimensionality of the inference problems, nonlinearity of the models and/or non-Gaussian statistics. Targeted application areas include the geoscience as well as emerging fields for data assimilation such as biophysics and cognitive neuroscience.
Speaker
Prof. Dr. Sebastian Reich, University of Potsdam, Institute of Mathematics
Managing Director
Lydia Stolpmann, University of Potsdam, Institute of Mathematics
News
Josie König successfully applied for a Fulbright Grant
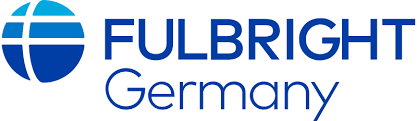
Congratulations to Josie König (PhD candidate in project A07) who succesfully applied for a Fulbright Germany Grant. With this Grant, Josie will… more ›
Focus Retreat on Hiddensee 2024
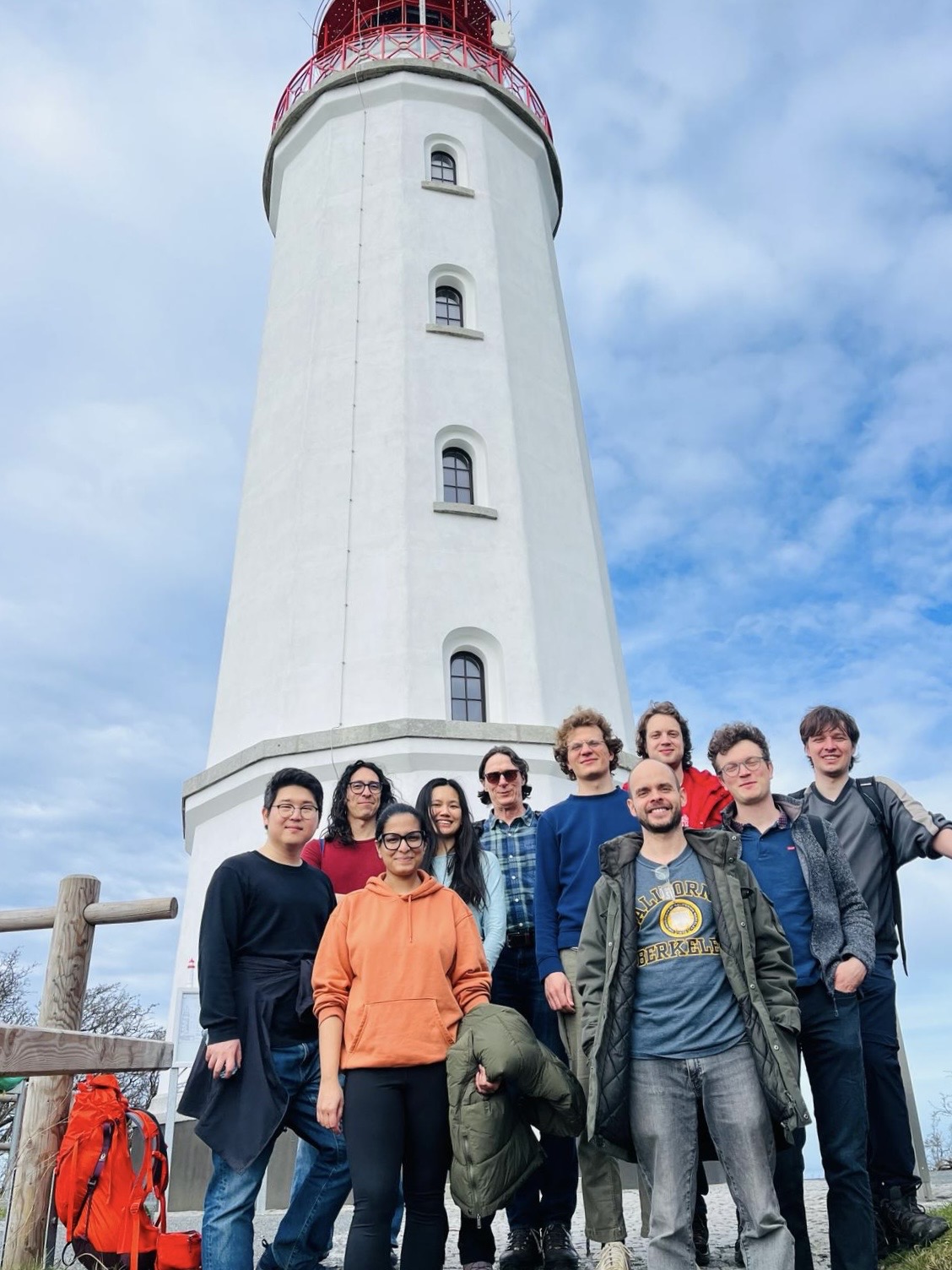
The 3rd Focus Retreat on Hiddensee took place from 4th to 8th of April, 2024. Sebastian Reich (Z01, A02, A06, B03, B09) invited Jan Albrecht (B07),… more ›
3rd IRTG Jamboree
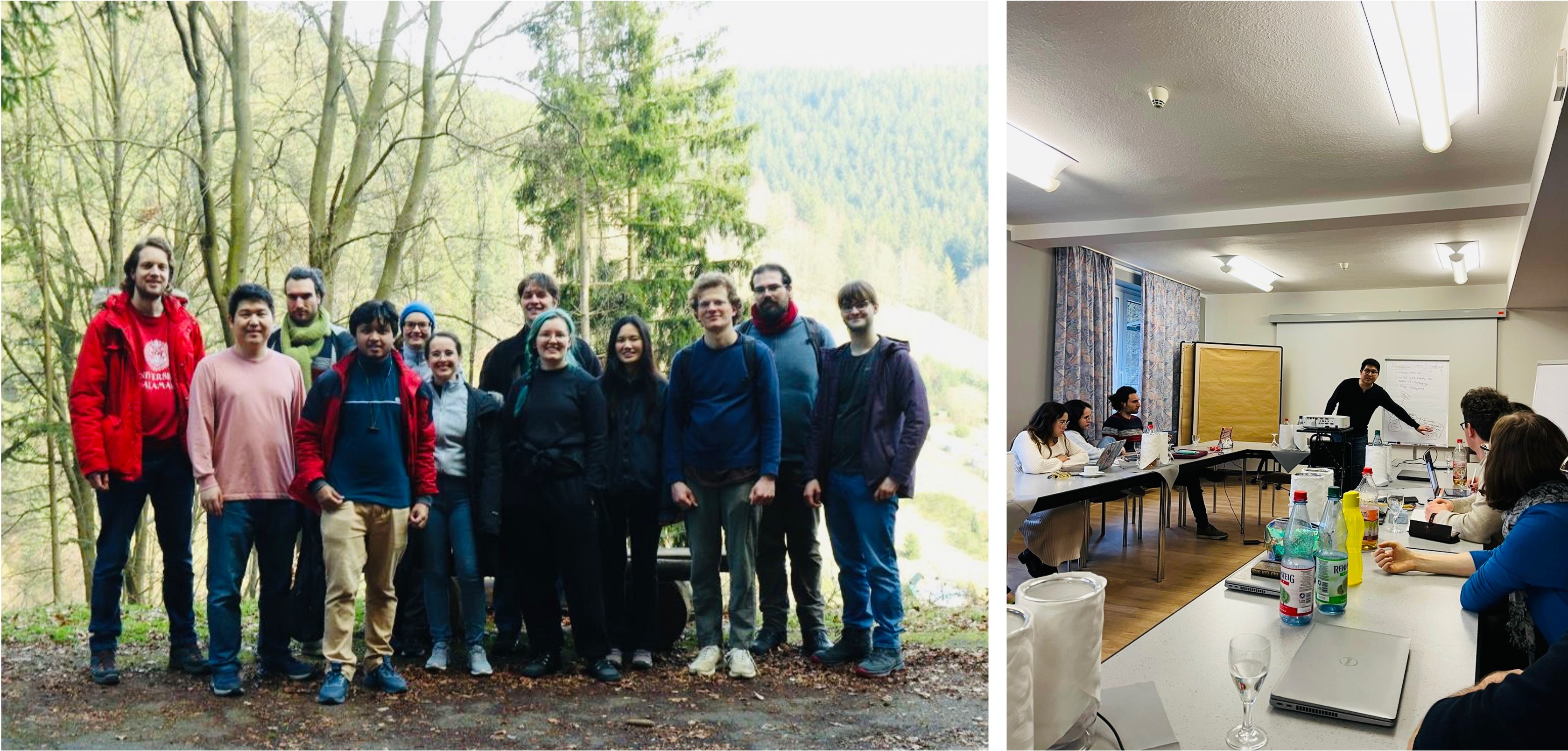
From March 13th to 15th, the PhD students and Postdocs of the IRTG as well as associated members traveled to Thuringia for their annual Jamboree. They… more ›
Upcoming Events
Kernel Methods in Generative Modeling
Gabriele Steidl, TU Berlin tba10:15 - 11:45
We consider gradient flows with respect to the maximum mean discrepancy (MMD) of certain kernels. For the efficient computation, we propose slicing…
more ›IRTG Workshop: Scientific Writing
Dr Martina Michalikova, Writing Scientist Golm09:00 - 17:00
Dr Martina Michalikova gives a two-day scientific writing workshop on the 6th and 7th of June 2024, from 9 a.m. - 5 p.m. The workshop focuses on…
more ›Random walks of heterogeneous population and ensemble self-reinforcement
Sergei Fedotov, University of Manchester 2.28.0.10810:15 - 11:45
The talk will be concerned with time-fractional master equations with random transition probabilities describing a heterogeneous population of random…
more ›Latest Publications
Haas, B., Shprits, Y. Y., Wutzig, M., Szabó-Roberts, M., García Peñaranda, M., Castillo Tibocha, A. M., Himmelsbach, J., Wang, D., Miyoshi, Y., Kasahara, S., Keika, K., Yokota, S., Shinohara, I., and Hori, T. (2024). Global validation of data-assimilative electron ring current nowcast for space weather applications.Sci Rep 14, 2327. doi.org/10.1038/s41598-024-52187-0.
Gottwald, G., Li, F., Marzouk, Y., Reich, S (2024). Stable generative modelling using diffusion maps. arXiv 2401.04372
König, J., Pfeffer, M. and Stoll, M. (2023). Efficient training of Gaussian processes with tensor product structure. arXiv 2312.15305.